SSLr v3: Using Cutting-Edge Deep Learning to Supercharge Object Detection
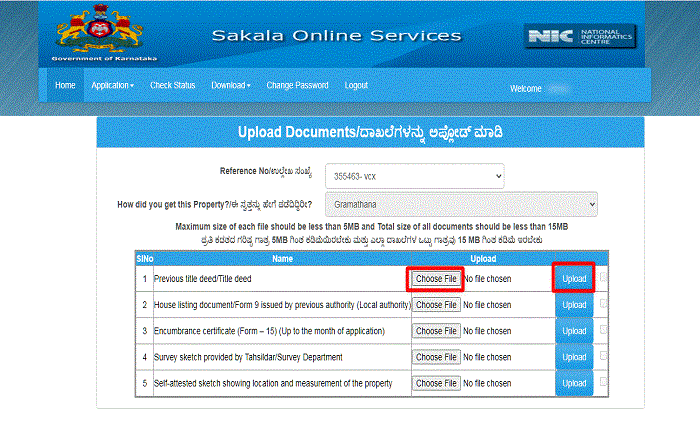
Thanks to deep learning’s capability, object detection has advanced significantly in the last several years. Cutting-edge models like YOLOv5 and Mask R-CNN have pushed the limits of efficiency and accuracy, opening up applications in fields like autonomous driving and medical imaging. Nonetheless, the pursuit of perpetually enhanced performance persists, and scientists are consistently investigating novel structures and methodologies to unleash more capabilities. This is where the state-of-the-art model SSLr v3, which is creating a lot of attention in the object detection field, comes into play.
Dissecting SSLr v3:
“SSLR with Transformers v3” is what SSLr v3 is short for. It improves upon the previous SSLr models, which pre-trained the model using a revolutionary self-supervised learning (SSL) technique. Using unlabeled data, SSL trains the model to anticipate associations between various visual components. This is a significant strategy to improve the model performance on small amounts of labelled data, as it enables the model to gain strong feature representations even in the absence of explicit object annotations.
The “v3” in SSLr v3 denotes the addition of multiple significant improvements over its predecessors. Among them are:
Transformer Encoder-Decoder design: The SSLr v3 employs a transformer encoder-decoder design, which is distinct from conventional convolutional neural networks (CNNs). This makes it possible for the model to extract features and comprehend objects more effectively by better capturing long-range dependencies within the image.
Hybrid Attention methods: The transformer encoder of SSLr v3 combines self-attention and channel attention methods. The model may prioritise relevant feature channels and concentrate on informative areas of the image with the help of self-attention and channel attention, which improves feature representation even further.
Dynamic Routing for Feature Fusion: Based on the unique image content, the model can now adaptively fuse features from several layers thanks to a dynamic routing method introduced in SSLr v3. As a result, features become more discriminative and contextually aware, increasing the accuracy of object detection.
SSLr v3’s advantages and benefits:
Compared to current object detection models, SSLr v3 has a number of strong advantages.
Superior Accuracy: On a variety of object identification datasets, extensive benchmark tests have shown that SSLr v3 performs better in terms of mean Average Precision (mAP) than other well-known models like Mask R-CNN and YOLOv5. It is perfect for jobs demanding extreme precision, including anomaly detection or medical picture analysis, because of its increased accuracy.
Efficiency and Speed: SSLr v3’s remarkable inference speed is maintained even with its complex architecture, which makes it appropriate for real-time applications such as autonomous driving and video monitoring. When using object detection models in contexts with limited resources, this efficiency is essential.
Decreased Data Dependency: Compared to previous models, SSLr v3 requires a lot less labelled data for fine-tuning because of its efficient pre-training using SSL. This is useful in situations when it is costly or time-consuming to gather huge amounts of labelled data.
Versatility and Adaptability: SSLr v3’s modular architecture allows for easy task adaptability to a range of object identification scenarios. Many applications are made possible by its transformer architecture, which makes it simple to integrate with other vision tasks like picture segmentation and classification.
Potential and Real-World Applications:
SSLr v3 has a wide range of potential applications in many domains.
Autonomous cars: SSLr v3 is perfect for object recognition in autonomous cars due to its excellent accuracy and real-time performance, which guarantees precise and safe navigation.
Medical Imaging: SSLr v3 can help physicians diagnose and plan treatments by accurately recognising suspicious lesions or tumours in medical pictures.
Automation and robotics: Providing robots with object identification capabilities enabled by SSLr v3 can improve their accuracy and safety when performing activities like item manipulation and navigation.
Retail & Security: Using SSLr v3 in security systems can significantly improve the accuracy of identifying shoplifters or suspicious activities in surveillance footage.
In summary:
SSLr v3 is proof of the ongoing development and innovation in the object detection space. Its distinctive combination of transformer design, SSL pre-training, and state-of-the-art attention mechanisms opens the door to unprecedented levels of precision, effectiveness, and adaptability. With additional research and development, SSLr v3 has the potential to completely transform object detection in a variety of fields, changing the way we use technology and traverse our environment.